EISM
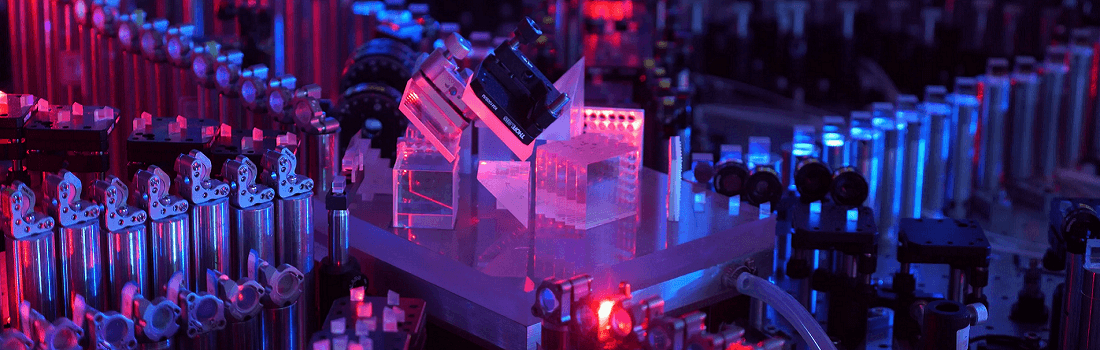
What is it?
As the name implies, Quantum AI is the use of quantum computing for computation of machine learning algorithms. Quantum computing and machine learning are both transformational technologies that will alter the way humans live and work. However, artificial intelligence is likely to require a deeper understanding of quantum computing and quantum mechanics before it achieves genuine human-like intelligence. In this regard, we believe EISM is at the cutting conceptual edge, drawing a symmetry between the information-processing associated with animal brains and the information-processing associated with the so-called “dS/dS” universe within string theory. To evaluate the basis of this claim, please see our research page or feel free to watch a public lecture here. The rest of this page is meant to give a basic overview of what Quantum AI is and why it is of particular interest to EISM.
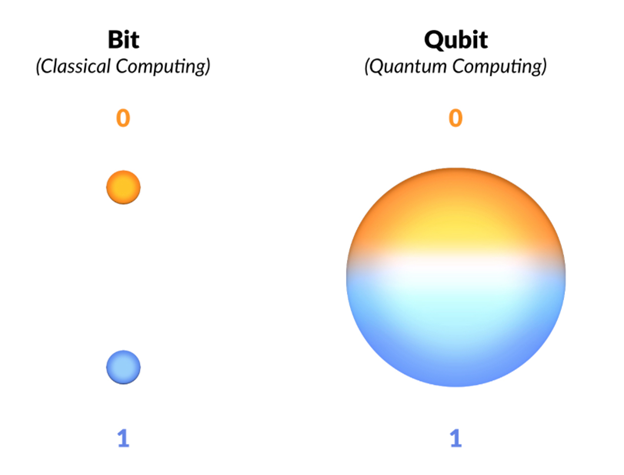
How does it work?
An ordinary computer works with 0s and 1s. Whatever task you ask it to perform, whether it’s calculating an equation or making a reservation, the underlying process is always the same: the task is translated into a string of 0s and 1s (the input), which is then processed by the computer. A new string of 0s and 1s emerges from the computer (the output), which gives you the result. Even if a computer program seems to work like magic, all it ever does is manipulate strings of bits — where each bit is either a 0 or a 1. On the machine level, this either/or dichotomy is represented using electrical circuits which can either be closed, in which case a current flows, or open, in which case there isn’t a current.
In contrast, quantum computing is based on the fact that, in the microscopic world, things act in a very peculiar way. Tiny particles, such as electrons or photons, can simultaneously take on states that we would normally deem mutually exclusive. They can be in several places at once, for example, and in the case of photons simultaneously exhibit two kinds of polarisation. This is the basis for Erwin Shrodinger’s famous cat:
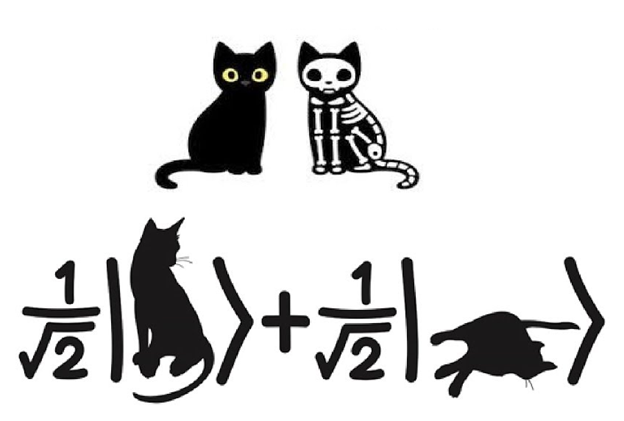
We never see this superposition of different states (such as a cat that is both dead and live at the same time) in ordinary life because this quantum effect disappears whenever a system is observed. For example, when we measure the location of an electron or the polarisation of a photon, all possibilities are eliminated and we see only one. Physicists in general are not do not know how this happens, but the work of Israeli physicist Yakir Aharanov strongly suggests a solution that he refers to as the “two-time” interpretation of quantum mechanics. We will have a lot more to say about this on our research page and in future updates. For now, we will simply say that this two-time interpretation has important implications for everything that matters to humans, not the least of which is the practical application of Quantum AI.
Potential Applications
Thanks to computational advantages of quantum computing, quantum AI can help achieve results that are not possible to achieve with classical computers.
- Quantum data: Quantum data can be considered as data packets contained in qubits for computerization. However, observing and storing quantum data is challenging because of the features that make it valuable which are superposition and entanglement. In addition, quantum data is noisy, it is necessary to apply a machine learning in the stage of analyzing and interpreting these data correctly.
- Hybrid quantum-classical models: It is highly possible to obtain meaningless data only when using quantum processors to generate quantum data. For this reason, a hybrid model emerges when it is powered by fast data processing mechanisms such as CPU and GPU, which are frequently used in the classical computer.
- Quantum algorithms: An algorithm is a sequence of steps that leads to the solution of a problem. In order to execute these steps on a device, one must use specific instruction sets that the device is designed to do so. Quantum computing introduces different instruction sets that are based on a completely different idea of execution when compared with classical computing. The aim of quantum algorithms is to use quantum effects like superposition and entanglement to get the solution faster.
Quantum computing can be used for the rapid training of machine learning models and to create optimized algorithms. An optimized and stable AI provided by quantum computing can complete years of analysis in a short time and lead to advances in technology. Neuromorphic cognitive models, adaptive machine learning, or reasoning under uncertainty are some fundamental challenges of today’s AI. Quantum AI is likely to lead to breakthroughs in these and other unforeseeable areas.
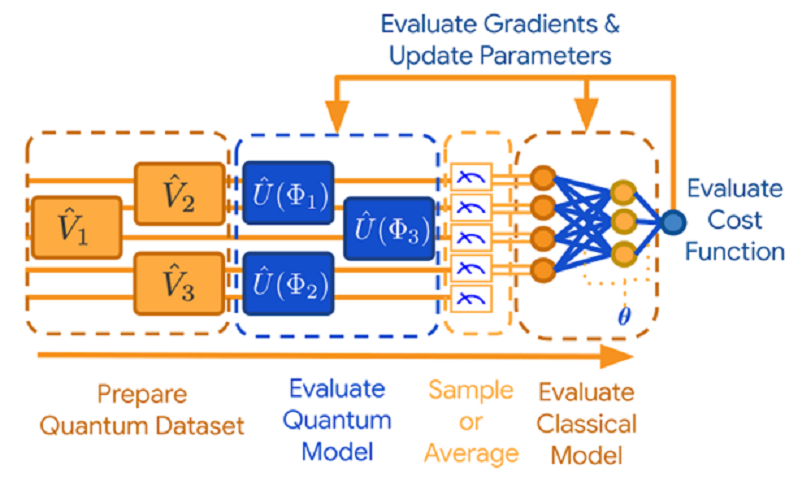
Recently, Google announced TensorFlow Quantum(TFQ): an open-source library for quantum machine learning. TFQ is designed to provide the necessary tools to control and model natural or artificial quantum systems. TFQ is an example of a suite of tools that combines quantum modeling and machine learning techniques.
Stay tuned for updates from EISM. We are on the cutting edge and will provide our customers with updates on the latest developments and their potential applications.