EISM
Natural language processing tools are important for organizations that work with large amounts of unstructured text such as emails, social media conversations, online chats, survey responses, etc. By incorporating NLP into the business process, companies can analyze data to gain valuable insights that help automate tasks and drive business decisions. As is the case in computer vision, NLP-related technology is having a disruptive impact on almost every industry. Here are some examples and use cases that EISM would be happy to think through with you:
Chatbots
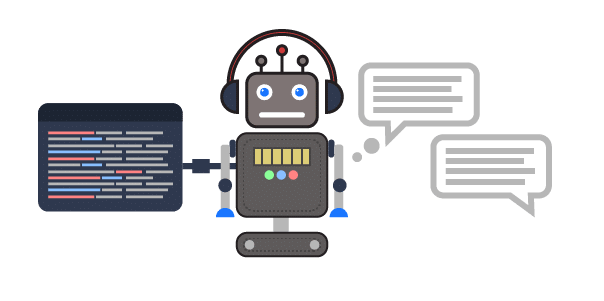
Chatbots are used to automate many aspects of customer service. They are designed to understand natural language and deliver an appropriate response through natural language generation.
In the past, most question-answering solutions followed pre-defined rules. In contrast, AI-powered chatbots and virtual assistants can learn from interactions and understand how to respond. What is especially valuable is the fact that the chatbots learn from experience and improve over time.
Chatbots are increasingly present at the frontline of customer support, as they can help teams solve a large percentage of routine queries while routing more complex issues to people. Given that they are available 24/7, chatbots and virtual assistants can speed up response times, and relieve agents from repetitive and time-consuming queries.
Text Classification
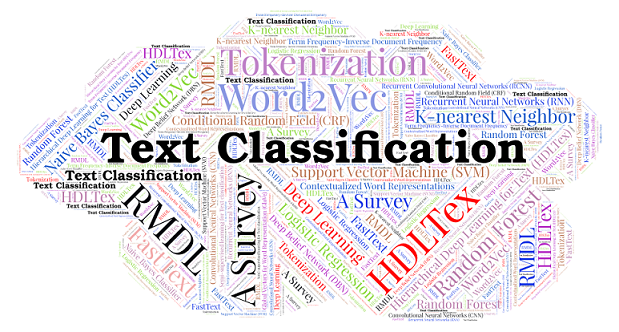
Text classification involves automatically understanding, processing, and categorizing unstructured text. For example, a common example is a person who needs to analyze hundreds of open-ended responses to a survey. Doing it manually would take a lot of time and end up being very expensive. But what if you could train a natural language processing model to automatically tag relevant data in just seconds, using predefined categories and applying an established criteria? It would save a lot of time and money.
For this kind of task, we can use a topic classifier for the survey responses. These automatically tag the data according to topics such as Customer Support, Features, Ease of Use, and Pricing.
If that sounds interesting, keep reading. There’s more value to be had from this technology.
Sentiment Analysis
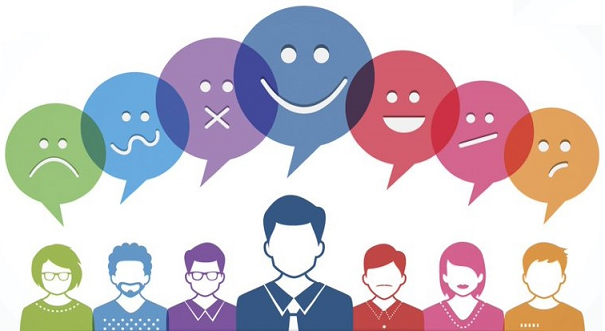
Natural human language understanding is especially difficult for machines when it comes to opinions, given the wide variety of sarcastic and ironic ideas that humans often need to convey. Sentiment analysis, however, is able to recognize subtle nuances in emotions and opinions ‒ and determine how positive or negative they are.
If we analyze sentiment in real-time, we can monitor mentions on social media and handle negative comments before they escalate. We can also gauge customer reactions to marketing campaigns and product launches. The end result is an effective and relatively inexpensive way to sense of how customers feel about our company.
You can also perform sentiment analysis periodically, and understand what customers like and dislike about specific aspects of your products and services ‒ maybe they love a new feature, but are disappointed about the customer service. These insights can help companies make smarter decisions because they show us exactly what needs to improve.
Market Intelligence
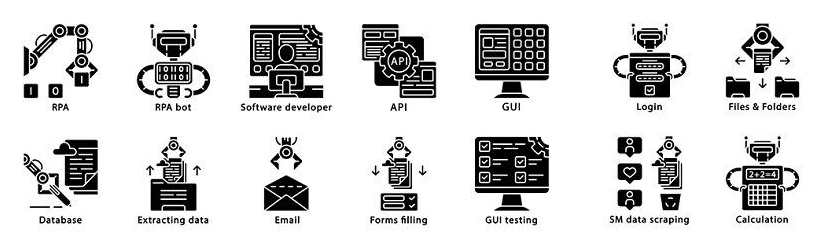
The role of machine learning in marketing is to allow you to quickly make decisions based on big data.
Traditionally, marketers create hypotheses, test them, evaluate them, and analyze them. This process is typically long and labor-intensive — and often the results are not useful because information changes so rapidly. By the time you finish the evaluation, things have changed either internally or in the market.
As a rough numerical example, to evaluate 20 advertising campaigns using 10 behavioral parameters for five different segments, a marketer will need, on average, about four hours. If this kind of analysis is an every day affair, then the marketer will spend about half of their time simply assessing the quality of campaigns. In contrast, when machine learning is used, evaluation takes minutes. Furthermore, the number of segments and behavior parameters is unlimited.
The fact is that with machine learning, we can respond faster to changes in the quality of traffic brought by our advertising campaigns. As a result, we can devote more time to creating hypotheses and verifying results rather than spending an inordinate and unnecessary amount of time on routine administrative actions. Machine learning makes marketers more effective and strategic, and that is always going to help businesses succeed.
If you are interested in any of the above examples and would like more information, please contact us. We would be happy to assess your situation and answer any questions you might have. If you would like to set up a Zoom meeting, click here. If you prefer to contact us through any other means, you will find our complete contact information here.