EISM
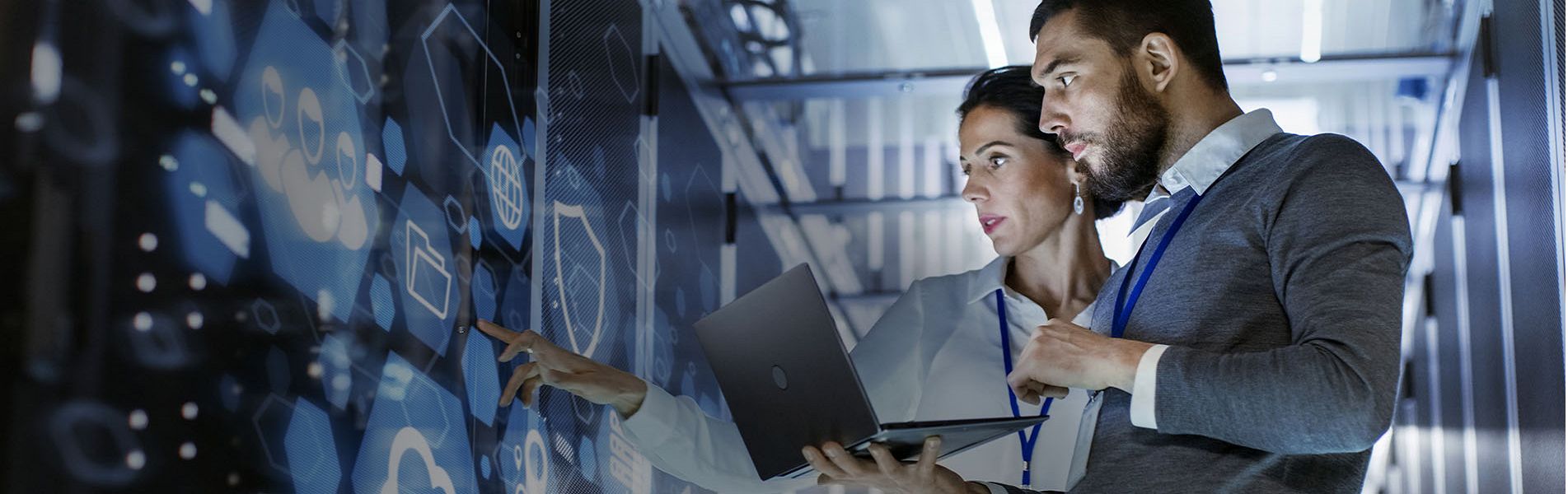
Sensors are the bread and butter of smart buildings. Much of the transformative technology changing the way we manage buildings relies on gathering ultra-accurate data—perhaps about room occupancy, temperature and air quality—and using it to automate processes or feeding it back to building managers. But this it just the beginning: the next generation of sensor-based devices will be able to analyse even richer data for even more accurate results, and it will do this using machine learning.
What is machine learning?
What is machine learning?
Machine learning involves using artificial intelligence to give a system the ability to improve itself by making observations, gathering data and learning from its experiences without being explicitly programmed or instructed to do so. It enables systems to analyse much larger quantities of data and deliver more accurate results. There are two major varieties of machine learning: supervised learning and unsupervised learning.In simple terms, supervised learning involves training the AI using ‘labelled’ data, i.e. data for which the correct answer is already known. For example, if you wanted to train an AI to manage the conditions of a meeting room, you would teach it that more lighting is required later in the day, or to order more coffee from the building management team for a more highly attended meeting. This takes significant time and expertise, but it ensures the algorithm is producing informed judgements.
Unsupervised learning involves allowing the AI to work on its own to discover information by drawing inferences from unlabelled data; for instance, by showing the AI a picture of a human it will learn to recognise the features that make humans distinct from other animals, and be able to identify one in the future based on those characteristics. Unsupervised learning is useful for finding unknown patterns in data—and therefore much more sophisticated predictions about—and eliminates time-consuming human supervision.
How will machine learning affect smart buildings?
How will machine learning affect smart buildings?
By making better decisions about heating and lighting usage based on the varying needs of a building’s occupants, machine learning can also improve energy management and therefore reduce a building’s carbon footprint. It has implication for smart cities, too. By aggregating data about vehicle flow, building occupancy and pedestrian numbers, cities can direct traffic in and around cities more efficiently, reducing congestion, and in turn, pollution. This is only possible, however, with widespread adoption of smart systems and machine learning by buildings through a city.
What does the future hold for machine learning?
What does the future hold for machine learning?
Furthermore, machines may still be unable to understand anomalous results: an unproductive employee whose performance doesn’t improve based on the lighting, heating or air quality of their workspace might instead be experiencing mental health problems, something the system is not trained to recognise.
These systems have huge benefits to building management, and they’re evolving fast. To keep up with these developments and discover how machine learning can be integrated into your building, register your interest for Intelligent Building Europe 2021, taking place on 18-20 May 2021.
Source: www.intelligentbuildingeurope.com.