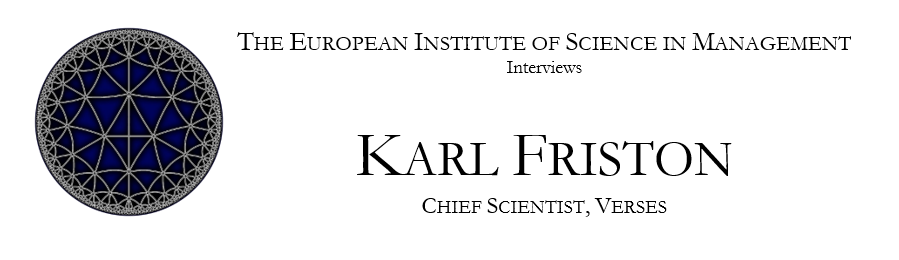
Karl Friston is a theoretical neuroscientist and world-renowned authority on brain imaging. He is also the Chief Scientist at Verses (NEO:VERS) (OTCQX:VRSSF). He invented statistical parametric mapping (SPM), voxel-based morphometry (VBM) and dynamic causal modelling (DCM). These contributions were motivated by schizophrenia research and theoretical studies of value-learning – formulated as the dysconnection hypothesis of schizophrenia. His main contribution to theoretical neurobiology is a free-energy principle for action and perception (active inference). Among his many accolades, Friston was awarded the Minerva Golden Brain Award and was elected a Fellow of the Royal Society in 2006. In 2016 he recieved the Charles Branch Award for unparalleled breakthroughs in Brain Research and the Glass Brain Award – a lifetime achievement award in the field of human brain mapping. He holds Honorary Doctorates from the universities of York, Zurich, Liège and Radboud University.
In this interview, Dr. Friston talks to Luis Razo Bravo, the executive director of EISM, about Friston’s recent appointment as Chief Scientist at Verses. Friston also talks about his recent paper on taking a first-principles physics approach to artificial general intelligence (AGI) and promoting long-term human survival through “collective intelligence”.
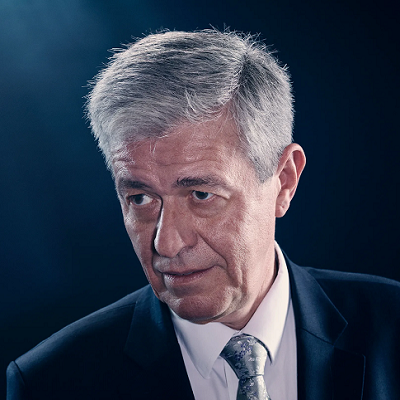
* The following text has been edited and paraphrased for clarity. To listen to the full interview, click on the video thumbnail below.
Professor Friston, can you tell us about your recent paper on the future of artificial general intelligence and your concept of “shared intelligence”?
First of all this technology, this white paper is the product of many months, if not years, of work by people like the head of research and development of Verses, people like Maxwell Ramstead and all his colleagues, with whom he has been in discussion with on the Verses side. So in the past few weeks really, to coincide with the announcement of my chief scientist appointment, it’s been brought to fruition and rendered in a way that is very clearly grounded in a first principles account of self-organization and intelligence.
For me the big thing was being forced to look at what the implication of this understanding of the way that we share beliefs and we interact with others, has for the future. So it was a very audacious white paper, rolling out decades into the future. The basic premise, there were lots of really interesting technical issues about how one will deploy these ideas and this technology and what is necessary for that deployment, practically, in terms of what people need to be doing in the next few years, on the one hand, and then on the other hand, there was the ultimate direction of travel.
The vision of the future and, for me, the more compelling aspect was this focus on a move from the information age to the age of intelligence, and especially the nature in which that intelligence is shared. So it’s all about communication. If you were in the neurosciences this would be a picture, a formal picture, of distributed cognition. From my perspective, it’s a realization of the fundaments of coupling to the world, exchanging messages in an optimal way that speaks to exactly this notion of how we share beliefs.
How does a “free-energy” or “active inference” approach to artificial general intelligence compare to conventional AI?
So the free energy principle and active inference distinguishes itself from most optimization schemes that one might find in say artificial intelligence research that are predicated on optimizing some well-defined value functional minimizing some well-defined cost function and takes us into the world of Bayesian beliefs probability distributions very much like quantum physics It’s all about the probabilistic description of the way things are and in that sense everything is a kind of measurement or influence, so technically it was a move from having information as the currency or technically finding functions that map from data to some desired outcome and reformulating that kind of artificial intelligence in terms of active inference in the free energy principle, which brings us into the space of beliefs. It brings us into a different kind of calculus and a different kind of geometry, something that some people would cast as a Bayesian mechanics to complement things like quantum mechanics and classical mechanics and statistical mechanics.
So that tells you immediately that if you’re talking about a web of intelligence, you’re now in the game of talking about the ways in which we share beliefs and communicate beliefs and that immediately brings you to some very practical issues about the nature of message passing. I look at this through the lens of a theoretician, on the one hand, but also in making those ideas accessible and providing proof of concept, one also has to put them in silico and write down equations and you’ll have software demos of this kind of approach. In so doing, you have to commit to how you’re going to do this kind of shared intelligence on a computer – how you’re going to do it in terms of the computational architecture, and that usually boils down to message passing on graphs, knowing that if the future is all about sharing our beliefs and resolving our uncertainty, imbuing different things with different kinds of confidence, then you know the messages have to be passed in a way that communicates probability distributions or beliefs, and you then can appeal to this first principles account of self-organizing systems that have to pass messages from themselves to their environment, to the network, to the nodes outside of themselves, and also receive them.
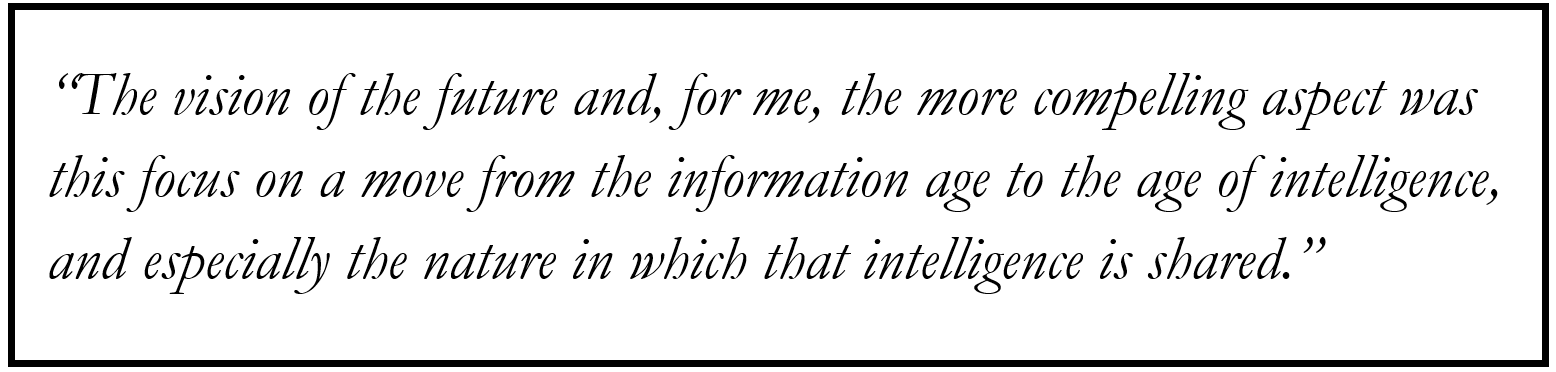
Can you explain the graphic that you often use involving a drop of ink and a drop of oil in water?
The graphic I typically use for this tries to give the intuition behind the physics of self-organization. As soon as you say the word self-organization, you immediately are committed to talking about itself as “something”, which means that you have to be able to distinguish the thing from everything else. Furthermore, that thing has to have characteristic states that are non-trivial in the sense that they can be measured or observed over a substantial period of time. So technically what we’re talking about are dynamical systems that self-organize to restrict themselves to an attracting set of states, and just defining thingness in terms of possessing an attracting set of States some characteristic States in which you would find me as some very simple um but also very telling consequences for the Dynamics of such things that exist in the sense of having these characteristic States.
So this is the graphic that I usually use just to try to demystify but also help people interpret the role of the mathematics that formalizes the dynamics in question. I ask people to imagine that I’m placing a drop of oil in a cup of water. What would normally happen in systems that don’t have characteristic states is that the water, that is, the ink molecules, would diffuse and dissipate throughout the solvent, due to random fluctuations at a molecular level. But the kind of systems that we’re interested in describing and characterizing are those which have an attracting set, as if, for example, the ink molecules gathered themselves together. In other words, that they were attracted to a particular location in this same glass of water. Just knowing that they do this tells you immediately that you can understand two forces that underwrite this aspect of self-organization. The first is the tendency for the ink molecules to dissipate or disperse or dissolve throughout the water, and that, I repeat, is due to the random fluctuations. This dissipation is exactly balanced by a gradient flow in the sense that the ink molecules look as if they are diffusing up concentration gradients. So when the diffusion up the concentration gradients exactly counters the dispersive effect of the random fluctuations then you get the emergence of this attracting set. That tells you immediately something fundamentally constructive about the dynamics of things that self-organize or simply exist: they are always moving up the log of the probability gradients that describe the states that they are characteristically in. And when you write that down you basically get the free energy principle.
Isaac Newton suspected that attraction and repulsion are central to all of nature. Can you explain the role that attraction and repulsion play in your Markovian blanket simulations?
In any kind of simulation under some sort of mild constraints, the role of these repulsions and attractions is simply to introduce a sparse coupling into a dynamical system so what we’re talking about here is the generic universal behavior of sparsely coupled dynamical systems and, in particular, random dynamical systems. So we have random fluctuations, so the equations of motion which were solved to produce this simulation of random fluctuations on them to provide a form of um stochastic chaos. So I emphasize the sparsity because that’s going to be very important in terms of understanding message passing and coupling, and certainly at a societal level. It certainly is extremely important in terms of the structure of our world.
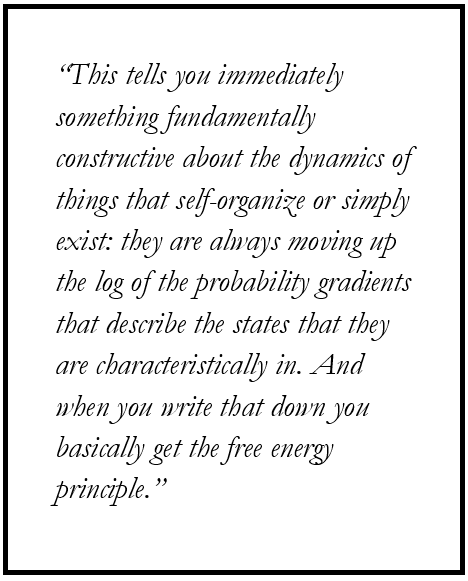
In this instance, we introduce sparsity implicitly by a certain locality of interactions. So if I have a number of nodes in a graph and form molecules and some idealized gas that only see their neighborhood but if you imagine a big connectivity Matrix or a coupling Matrix that couples every element of this population to every other member of the population then that locality forces all the connectivity to be along the leading diagonal and therefore you can say it to have sparse coupling because most of the allowable possible connections are non-existent so very much like many things in our lived world and the local coupling introduces a particular sparsity structure on your causal architecture that has important implications when it comes to carving nature at its joints in individuating certain parts of the system from other parts of other parts of the system.
In your quote of Newton, we would all acknowledge that he was absolutely right. I mean to carve nature at its joints, if those joints are articulated in terms of the way things couple to each other, you know everything should be derived from that coupling. And, of course, if everything was attractive, everything would be attracted to a point attractor, which would be not very interesting. And if everything was repulsive, there wouldn’t be an attractor, so there has to be attraction and repulsion to get these attracting sets. I hadn’t seen that quote of Newton before but it’s very impressive.
We talked, way too quickly, about the connection between active inference and voting, so we’ll have to talk again. In the meantime, what are the next steps for you?
Yes, I can see we’re going to have to continue this conversation. I’m just thinking your last summary was the same for me. I mean I just wanted to comment, perhaps it’s a thing we could develop in a few months’ time. That last treatment about voting and social choice theory and your population dynamics and the spread of ideas and that kind of thing. You framed it in terms of what is the agenda and you used a phrase which I thought was quite important, where you were likening the ultimate agenda to a kind of equilibrium and I would imagine part of that would be a synchronicity and a synchronization and a harmony. I think that’s so important you know and just as a layman now, you know it seems that that specification of the direction of travel of what is a good thing to do is very important. It is not growth. It is sustainability. The aspiration is not to move away or to have, you know, the slope going up forever but to keep things at equilibrium, which is of course one expression of the dangers of inequality.
That’s something we should talk about, my point being of course that you know as a physicist this all starts with how on earth can I describe a system that aspires to a non-equilibrium steady state? So it is this steady state aspect which is at the heart of all of this physics that we’ve been talking about and it’s nice that your ultimate agenda highlights and identifies exactly the same phenomena.
This has implications in terms of being able to detect fake news, for example. So all of these are really important aspects of the of the mechanics that you would associate or would need to understand to formalize distributed cognition or shared intelligence. I’m not saying that’s what I’m going to do next, but that’s certainly what I would like to do. Those are the areas I think present the immediate challenges.
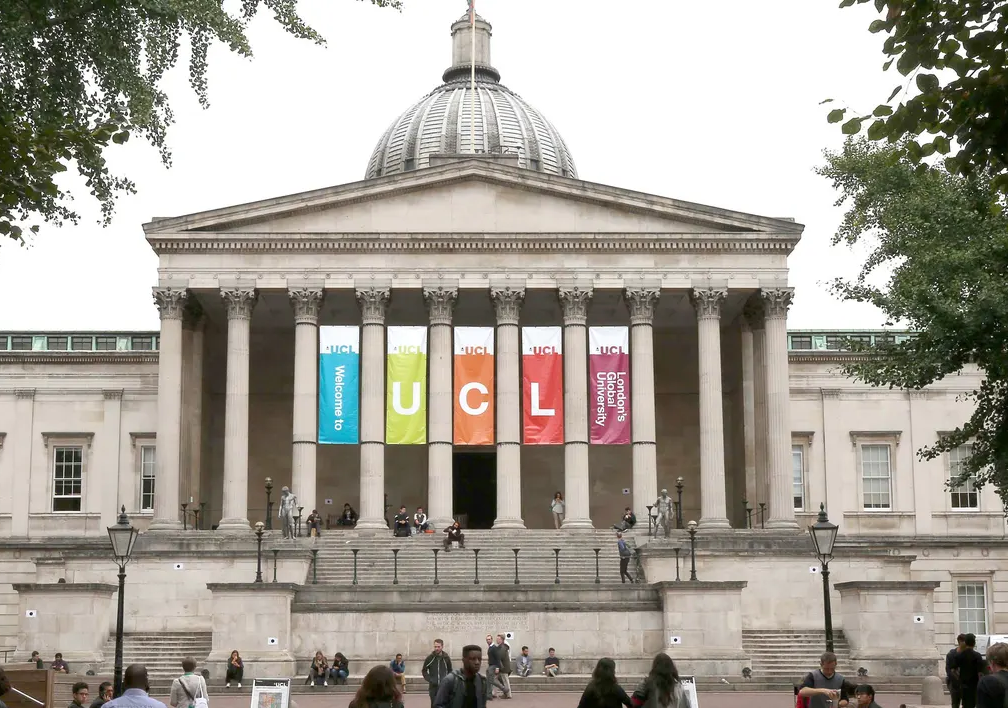
Another key point that you were figuring out when you were manipulating the number of votes per person, I was thinking about basically the precision error, you know, the question of how much weight you prescribe to these votes. That’s going to be a really crucial thing. Who should I listen to?